NLU vs NLP: AI Language Processing’s Unknown Secrets

Both of these technologies are beneficial to companies in various industries. NLU is used in a variety of applications, including virtual assistants, chatbots, and voice assistants. These systems use NLU to understand the user’s input and generate a response that is tailored to their needs. For example, a virtual assistant might use NLU to understand a user’s request to book a flight and then generate a response that includes flight options and pricing information. The computational methods used in machine learning result in a lack of transparency into “what” and “how” the machines learn.
Semantic Role Labeling (SRL) is a pivotal tool for discerning relationships and functions of words or phrases concerning a specific predicate in a sentence. This nuanced approach facilitates more nuanced and contextually accurate language interpretation by systems. NLP is a field that deals with the interactions between computers and human languages. It’s aim is to make computers interpret natural human language in order to understand it and take appropriate actions based on what they have learned about it. Natural language processing (NLP) and natural language understanding(NLU) are two cornerstones of artificial intelligence.

NLP is an interdisciplinary field that combines multiple techniques from linguistics, computer science, AI, and statistics to enable machines to understand, interpret, and generate human language. In human language processing, NLP and NLU, while visually resembling each other, serve distinct functions. Examining “NLU vs NLP” reveals key differences in four crucial areas, highlighting the nuanced disparities between these technologies in language interpretation. Data pre-processing aims to divide the natural language content into smaller, simpler sections.
How do NLU and NLP interact?
It considers the surrounding words, phrases, and sentences to derive meaning and interpret the intended message. Language generation is used for automated content, personalized suggestions, virtual assistants, and more. Systems can improve user experience and communication by using NLP’s language generation. Information retrieval, question-answering systems, sentiment analysis, and text summarization utilise NER-extracted data. NER improves text comprehension and information analysis by detecting and classifying named things.
It is a way that enables interaction between a computer and a human in a way like humans do using natural languages like English, French, Hindi etc. Conversational interfaces are powered primarily by natural language processing (NLP), and a key subset of NLP is natural language understanding (NLU). The terms NLP and NLU are often used interchangeably, but they have slightly different meanings. Developers need to understand the difference between natural language processing and natural language understanding so they can build successful conversational applications. NLU focuses on understanding the meaning and intent of human language, while NLP encompasses a broader range of language processing tasks, including translation, summarization, and text generation.

Chatbots powered by NLP and NLU can understand user intents, respond contextually, and provide personalized assistance. Natural Language Generation (NLG) is an essential component of Natural Language Processing (NLP) that complements the capabilities of natural language understanding. While NLU focuses on interpreting human language, NLG takes structured and unstructured data and generates human-like language in response.
Now, consider that this task is even more difficult for machines, which cannot understand human language in its natural form. Natural Language Processing(NLP) is a subset of Artificial intelligence which involves communication between a human and a machine using a natural language than a coded or byte language. It provides the ability to give instructions to machines in a more easy and efficient manner. Businesses can benefit from NLU and NLP by improving customer interactions, automating processes, gaining insights from textual data, and enhancing decision-making based on language-based analysis. Customer feedback, brand monitoring, market research, and social media analytics use sentiment analysis. It reveals public opinion, customer satisfaction, and sentiment toward products, services, or issues.
More from Artificial intelligence
NLP, with its focus on language structure and statistical patterns, enables machines to analyze, manipulate, and generate human language. It provides the foundation for tasks such as text tokenization, part-of-speech tagging, syntactic parsing, and machine translation. NLP algorithms excel at processing and understanding the form and structure of language. This involves breaking down sentences, identifying grammatical structures, recognizing entities and relationships, and extracting meaningful information from text or speech data. NLP algorithms use statistical models, machine learning, and linguistic rules to analyze and understand human language patterns. Natural language processing is a subset of AI, and it involves programming computers to process massive volumes of language data.
As a result, algorithms search for associations and correlations to infer what the sentence’s most likely meaning is rather than understanding the genuine meaning of human languages. For machines, human language, also referred to as natural language, is how humans communicate—most often in the form of text. It comprises the majority of enterprise data and includes everything from text contained in email, to PDFs and other document types, chatbot dialog, social media, etc. The future of language processing and understanding is filled with limitless possibilities in the realm of artificial intelligence. Advancements in Natural Language Processing (NLP) and Natural Language Understanding (NLU) are revolutionizing how machines comprehend and interact with human language. NLP systems learn language syntax through part-of-speech tagging and parsing.
Transformer-based LLMs trained on huge volumes of data can autonomously predict the next contextually relevant token in a sentence with an exceptionally high degree of accuracy. NLP centers on processing and manipulating language for machines to understand, interpret, and generate natural language, emphasizing human-computer interactions. Its core objective is furnishing computers with methods and algorithms for effective processing and modification of spoken or written language. NLP primarily handles fundamental functions such as Part-of-Speech (POS) tagging and tokenization, laying the groundwork for more advanced language-related tasks within the realm of human-machine communication.
Constituency parsing combines words into phrases, while dependency parsing shows grammatical dependencies. NLP systems extract subject-verb-object relationships and noun phrases using parsing and grammatical analysis. Complex languages with compound words or agglutinative structures benefit from tokenization. By splitting text into smaller parts, following processing steps can treat each token separately, collecting valuable information and patterns. With the LENSai, researchers can now choose to launch their research by searching for a specific biological sequence. Or they may search in the scientific literature with a general exploratory hypothesis related to a particular biological domain, phenomenon, or function.
NLP provides the foundation for NLU by extracting structural information from text or speech, while NLU enriches NLP by inferring meaning, context, and intentions. This collaboration enables machines to not only process and generate human-like language but also understand and respond intelligently to user inputs. NER uses contextual information, language patterns, and machine learning algorithms to improve entity recognition accuracy beyond keyword matching. NER systems are trained on vast datasets of named items in multiple contexts to identify similar entities in new text.
NLU is at the forefront of advancements in AI and has the potential to revolutionize areas such as customer service, personal assistants, content analysis, and more. One of the primary goals of NLP is to bridge the gap between human communication and computer understanding. By analyzing the structure and meaning of language, NLP aims to teach machines to process and interpret natural language in a way that captures its nuances and complexities. NLP relies on syntactic and structural analysis to understand the grammatical composition of texts and phrases. By focusing on surface-level inspection, NLP enables machines to identify the basic structure and constituent elements of language. This initial step facilitates subsequent processing and structural analysis, providing the foundation for the machine to comprehend and interact with the linguistic aspects of the input data.
In conclusion, I hope now you have a better understanding of the key differences between NLU and NLP. This will empower your journey with confidence that you are using both terms in the correct context. Whether it’s simple chatbots or sophisticated AI assistants, NLP is an integral part of the conversational app building process. And the difference between NLP and NLU is important to remember when building a conversational app because it impacts how well the app interprets what was said and meant by users. NLU seeks to identify the underlying intent or purpose behind a given piece of text or speech. It classifies the user’s intention, whether it is a request for information, a command, a question, or an expression of sentiment.
The main objective of NLU is to enable machines to grasp the nuances of human language, including context, semantics, and intent. It involves various tasks such as entity recognition, named entity recognition, sentiment analysis, and language classification. NLU algorithms leverage techniques like semantic analysis, syntactic parsing, and machine learning to extract relevant information from text or speech data and infer the underlying meaning. On the other hand, NLU goes beyond simply processing language to actually understanding it. NLU enables computers to comprehend the meaning behind human language and extract relevant information from text.
In machine learning (ML) jargon, the series of steps taken are called data pre-processing. The idea is to break down the natural language text into smaller and more manageable chunks. These can then be analyzed by ML algorithms to find relations, dependencies, and context among various chunks. NLU enables machines to understand and interpret human language, while NLG allows machines to communicate back in a way that is more natural and user-friendly.
It also facilitates sentiment analysis, which involves determining the sentiment or emotion expressed in a piece of text, and information retrieval, where machines retrieve relevant information based on user queries. NLP has the potential to revolutionize industries such as healthcare, customer service, information retrieval, and language education, among others. NLP employs both rule-based systems and statistical models to analyze and generate text. Linguistic patterns and norms guide rule-based approaches, where experts manually craft rules for handling language components like syntax and grammar.
The two most common approaches are machine learning and symbolic or knowledge-based AI, but organizations are increasingly using a hybrid approach to take advantage of the best capabilities that each has to offer. In addition to processing natural language similarly to a human, NLG-trained machines are now able to generate new natural language text—as if written by another human. All this has sparked a lot of interest both from commercial adoption and academics, making NLP one of the most active research topics in AI today. According to various industry estimates only about 20% of data collected is structured data. The remaining 80% is unstructured data—the majority of which is unstructured text data that’s unusable for traditional methods.
NLP systems can extract subject-verb-object relationships, verb semantics, and text meaning from semantic analysis. Information extraction, question-answering, and sentiment analysis require this data. Join us as we unravel the mysteries and unlock the true potential of language processing in AI. Modern NLP systems are powered by three distinct natural language technologies (NLT), NLP, NLU, and NLG. It takes a combination of all these technologies to convert unstructured data into actionable information that can drive insights, decisions, and actions.
To explore the exciting possibilities of AI and Machine Learning based on language, it’s important to grasp the basics of Natural Language Processing (NLP). It’s like taking the first step into a whole new world of language-based technology. Furthermore, based on specific use cases, we will investigate the scenarios in which favoring one skill over the other becomes more profitable for organizations. This research will provide you with the insights you need to determine which AI solutions are most suited to your organization’s specific needs.
NLP primarily focuses on surface-level aspects such as sentence structure, word order, and basic syntax. You can foun additiona information about ai customer service and artificial intelligence and NLP. However, its emphasis is limited to language processing and manipulation without delving deeply into the underlying semantic layers of text or voice data. NLP excels in tasks related to the structural aspects of language but doesn’t extend its reach to a profound understanding of the nuanced meanings or semantics within the content. Instead, machines must know the definitions of words and sentence structure, along with syntax, sentiment and intent.
NLP full form is Natural Language Processing (NLP) is an exciting field that focuses on enabling computers to understand and interact with human language. It involves the development of algorithms and techniques that allow machines to read, interpret, and respond to text or speech in a way that resembles human comprehension. Natural Language Understanding (NLU), a subset of Natural Language Processing (NLP), employs semantic analysis to derive meaning from textual content. NLU addresses the complexities of language, acknowledging that a single text or word may carry multiple meanings, and meaning can shift with context. Through computational techniques, NLU algorithms process text from diverse sources, ranging from basic sentence comprehension to nuanced interpretation of conversations.
Use Cases for Graph Databases
NLP models can determine text sentiment—positive, negative, or neutral—using several methods. This analysis helps analyze public opinion, client feedback, difference between nlp and nlu social media sentiments, and other textual communication. Natural Language Processing (NLP) relies on semantic analysis to decipher text.
Tokenization, part-of-speech tagging, syntactic parsing, machine translation, etc. Parsing and grammatical analysis help NLP grasp text structure and relationships. Parsing establishes sentence hierarchy, while part-of-speech tagging categorizes words. 4 min read – As AI transforms and redefines how businesses operate and how customers interact with them, trust in technology must be built. The verb that precedes it, swimming, provides additional context to the reader, allowing us to conclude that we are referring to the flow of water in the ocean. The noun it describes, version, denotes multiple iterations of a report, enabling us to determine that we are referring to the most up-to-date status of a file.
Over 50 years later, human language technologies have evolved significantly beyond the basic pattern-matching and substitution methodologies that powered ELIZA. It deals with tasks like text generation, translation, and sentiment analysis. NLP helps computers understand and interpret human language by breaking down sentences into smaller parts, identifying words and their meanings, and analyzing the structure of language. For example, NLP can be used in chatbots to understand user queries and provide appropriate responses. The future of language processing and understanding with artificial intelligence is brimming with possibilities.
A key difference is that NLU focuses on the meaning of the text and NLP focuses more on the structure of the text. Our open source conversational AI platform includes NLU, and you can customize your pipeline in a modular way to extend the built-in functionality of Rasa’s NLU models. You can learn more about custom NLU components in the developer documentation, and be sure to check out this detailed tutorial. An example of NLU in action is a virtual assistant understanding and responding to a user’s spoken request, such as providing weather information or setting a reminder. As we embrace this future, responsible development and collaboration among academia, industry, and regulators are crucial for shaping the ethical and transparent use of language-based AI.
The platform also leverages the latest development in LLMs to bridge the gap between syntax (sequences) and semantics (functions). Natural language generation is another subset of natural language processing. While natural language understanding focuses on computer reading comprehension, natural language generation enables computers to write. NLG is the process of producing a human language text response based on some data input. This text can also be converted into a speech format through text-to-speech services.
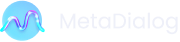
It goes beyond the structural aspects and aims to comprehend the meaning, intent, and nuances behind human communication. NLU tasks involve entity recognition, intent recognition, sentiment analysis, and contextual understanding. By leveraging machine learning and semantic analysis techniques, NLU enables machines to grasp the intricacies of human Chat PG language. By combining contextual understanding, intent recognition, entity recognition, and sentiment analysis, NLU enables machines to comprehend and interpret human language in a meaningful way. This understanding opens up possibilities for various applications, such as virtual assistants, chatbots, and intelligent customer service systems.
Understanding the Detailed Comparison of NLU vs NLP delves into their symbiotic dance, unveiling the future of intelligent communication. NLG also encompasses text summarization capabilities that generate summaries from in-put documents while maintaining the integrity of the information. Extractive summarization is the AI innovation powering Key Point Analysis used in That’s Debatable. To learn about the future expectations regarding NLP you can read our Top 5 Expectations Regarding the Future of NLP article. Chrissy Kidd is a writer and editor who makes sense of theories and new developments in technology.
- NLP is a broad field that encompasses a wide range of technologies and techniques, while NLU is a subset of NLP that focuses on a specific task.
- Extractive summarization is the AI innovation powering Key Point Analysis used in That’s Debatable.
- While natural language understanding focuses on computer reading comprehension, natural language generation enables computers to write.
- One of the primary goals of NLU is to teach machines how to interpret and understand language inputted by humans.
- When an unfortunate incident occurs, customers file a claim to seek compensation.
As seen in Figure 3, Google translates the Turkish proverb “Damlaya damlaya göl olur.” as “Drop by drop, it becomes a lake.” This is an exact word by word translation of the sentence. Using symbolic AI, everything is visible, understandable and explained within a transparent box that delivers complete insight into how the logic was derived. This transparency makes symbolic AI an appealing choice for those who want the flexibility to change the rules in their NLP model.
Natural language processing works by taking unstructured data and converting it into a structured data format. For example, the suffix -ed on a word, like called, indicates past tense, but it has the same base infinitive (to call) as the present tense verb calling. On the other hand, NLU delves deeper into the semantic understanding and contextual interpretation of language.
These approaches are also commonly used in data mining to understand consumer attitudes. In particular, sentiment analysis enables brands to monitor their customer feedback more closely, allowing them to cluster positive and negative social media comments and track net promoter scores. By reviewing comments with negative sentiment, companies are able to https://chat.openai.com/ identify and address potential problem areas within their products or services more quickly. Have you ever wondered how Alexa, ChatGPT, or a customer care chatbot can understand your spoken or written comment and respond appropriately? NLP and NLU, two subfields of artificial intelligence (AI), facilitate understanding and responding to human language.
And if the assistant doesn’t understand what the user means, it won’t respond appropriately or at all in some cases. NLU is also utilized in sentiment analysis to gauge customer opinions, feedback, and emotions from text data. Additionally, it facilitates language understanding in voice-controlled devices, making them more intuitive and user-friendly.
For instance, inflated statements and an excessive amount of punctuation may indicate a fraudulent review. Questionnaires about people’s habits and health problems are insightful while making diagnoses. In this section, we will introduce the top 10 use cases, of which five are related to pure NLP capabilities and the remaining five need for NLU to assist computers in efficiently automating these use cases.
Language processing begins with tokenization, which breaks the input into smaller pieces. Tokens can be words, characters, or subwords, depending on the tokenization technique. The search-based approach uses a free text search bar for typing queries which are then matched to information in different databases. A key limitation of this approach is that it requires users to have enough information about the data to frame the right questions.
NLU goes beyond literal interpretation and involves understanding implicit information and drawing inferences. It takes into account the broader context and prior knowledge to comprehend the meaning behind the ambiguous or indirect language. This allows computers to summarize content, translate, and respond to chatbots. Sometimes people know what they are looking for but do not know the exact name of the good. In such cases, salespeople in the physical stores used to solve our problem and recommended us a suitable product. In the age of conversational commerce, such a task is done by sales chatbots that understand user intent and help customers to discover a suitable product for them via natural language (see Figure 6).
They enable computers to analyse the meaning of text and spoken sentences, allowing them to understand the intent behind human communication. NLP is the specific type of AI that analyses written text, while NLU refers specifically to its application in speech recognition software. NLU extends beyond basic language processing, aiming to grasp and interpret meaning from speech or text. Its primary objective is to empower machines with human-like language comprehension — enabling them to read between the lines, deduce context, and generate intelligent responses akin to human understanding. NLU tackles sophisticated tasks like identifying intent, conducting semantic analysis, and resolving coreference, contributing to machines’ ability to engage with language at a nuanced and advanced level.
It’s a subset of NLP and It works within it to assign structure, rules and logic to language so machines can “understand” what is being conveyed in the words, phrases and sentences in text. NLU is the final step in NLP that involves a machine learning process to create an automated system capable of interpreting human input. This requires creating a model that has been trained on labelled training data, including what is being said, who said it and when they said it (the context). NLU is widely used in virtual assistants, chatbots, and customer support systems. NLP finds applications in machine translation, text analysis, sentiment analysis, and document classification, among others.
NLP, with its ability to identify and manipulate the structure of language, is indeed a powerful tool. By considering clients’ habits and hobbies, nowadays chatbots recommend holiday packages to customers (see Figure 8). Before booking a hotel, customers want to learn more about the potential accommodations. People start asking questions about the pool, dinner service, towels, and other things as a result. Such tasks can be automated by an NLP-driven hospitality chatbot (see Figure 7). Sentiment analysis, thus NLU, can locate fraudulent reviews by identifying the text’s emotional character.
It involves tasks such as semantic analysis, entity recognition, and language understanding in context. NLU aims to bridge the gap between human communication and machine understanding by enabling computers to grasp the nuances of language and interpret it accurately. For instance, NLU can help virtual assistants like Siri or Alexa understand user commands and perform tasks accordingly. It extracts pertinent details, infers context, and draws meaningful conclusions from speech or text data. While delving deeper into semantic and contextual understanding, NLU builds upon the foundational principles of natural language processing.
NLU is a subset of NLP that focuses on understanding the meaning of natural language input. NLU systems use a combination of machine learning and natural language processing techniques to analyze text and speech and extract meaning from it. The power of collaboration between NLP and NLU lies in their complementary strengths. While NLP focuses on language structures and patterns, NLU dives into the semantic understanding of language. Together, they create a robust framework for language processing, enabling machines to comprehend, generate, and interact with human language in a more natural and intelligent manner.
And if we decide to code rules for each and every combination of words in any natural language to help a machine understand, then things will get very complicated very quickly. When it comes to natural language, what was written or spoken may not be what was meant. In the most basic terms, NLP looks at what was said, and NLU looks at what was meant. People can say identical things in numerous ways, and they may make mistakes when writing or speaking.
NLG, on the other hand, is a field of AI that focuses on generating natural language output. While both these technologies are useful to developers, NLU is a subset of NLP. This means that while all natural language understanding systems use natural language processing techniques, not every natural language processing system can be considered a natural language understanding one. This is because most models developed aren’t meant to answer semantic questions but rather predict user intent or classify documents into various categories (such as spam).
One of the primary goals of NLU is to teach machines how to interpret and understand language inputted by humans. NLU leverages AI algorithms to recognize attributes of language such as sentiment, semantics, context, and intent. It enables computers to understand the subtleties and variations of language. For example, the questions “what’s the weather like outside?” and “how’s the weather?” are both asking the same thing. The question “what’s the weather like outside?” can be asked in hundreds of ways. With NLU, computer applications can recognize the many variations in which humans say the same things.
NLU & NLP: AI’s Game Changers in Customer Interaction – CMSWire
NLU & NLP: AI’s Game Changers in Customer Interaction.
Posted: Fri, 16 Feb 2024 08:00:00 GMT [source]
Natural language processing and its subsets have numerous practical applications within today’s world, like healthcare diagnoses or online customer service. Machine learning uses computational methods to train models on data and adjust (and ideally, improve) its methods as more data is processed. Grammar complexity and verb irregularity are just a few of the challenges that learners encounter.
NLU allows computer applications to infer intent from language even when the written or spoken language is flawed. As NLP algorithms become more sophisticated, chatbots and virtual assistants are providing seamless and natural interactions. Meanwhile, improving NLU capabilities enable voice assistants to understand user queries more accurately. Entity recognition, intent recognition, sentiment analysis, contextual understanding, etc. By harnessing advanced algorithms, NLG systems transform data into coherent and contextually relevant text or speech.

Hybrid natural language understanding platforms combine multiple approaches—machine learning, deep learning, LLMs and symbolic or knowledge-based AI. They improve the accuracy, scalability and performance of NLP, NLU and NLG technologies. The combination of NLP and NLU has revolutionized various applications, such as chatbots, voice assistants, sentiment analysis systems, and automated language translation.
NLP can process text from grammar, structure, typo, and point of view—but it will be NLU that will help the machine infer the intent behind the language text. So, even though there are many overlaps between NLP and NLU, this differentiation sets them distinctly apart. Latin, English, Spanish, and many other spoken languages are all languages that evolved naturally over time. Harness the power of artificial intelligence and unlock new possibilities for growth and innovation. Our AI development services can help you build cutting-edge solutions tailored to your unique needs. Whether it’s NLP, NLU, or other AI technologies, our expert team is here to assist you.